Featured Acticle
lobal Rice Multi-Class Segmentation Dataset (RiceSEG): A Comprehensive and Diverse Collection of High-Resolution RGB-Annotated Images for the Development and Benchmarking of Rice Segmentation Algorithms
Abstract
Developing computer vision–based rice phenotyping techniques is crucial for precision field management and accelerating breeding, thereby continuously advancing rice production. Among phenotyping tasks, distinguishing image components is a key prerequisite for characterizing plant growth and development at the organ scale, enabling deeper insights into eco-physiological processes. However, due to the fine structure of rice organs and complex illumination within the canopy, this task remains highly challenging, underscoring the need for a high-quality training dataset. Such datasets are scarce, both due to a lack of large, representative collections of rice field images and the time-intensive nature of annotation. To address this gap, we established the first comprehensive multi-class rice semantic segmentation dataset, RiceSEG. We gathered nearly 50,000 high-resolution, ground-based images from five major rice-growing countries (China, Japan, India, the Philippines, and Tanzania), encompassing over 6,000 genotypes across all growth stages...
Under Review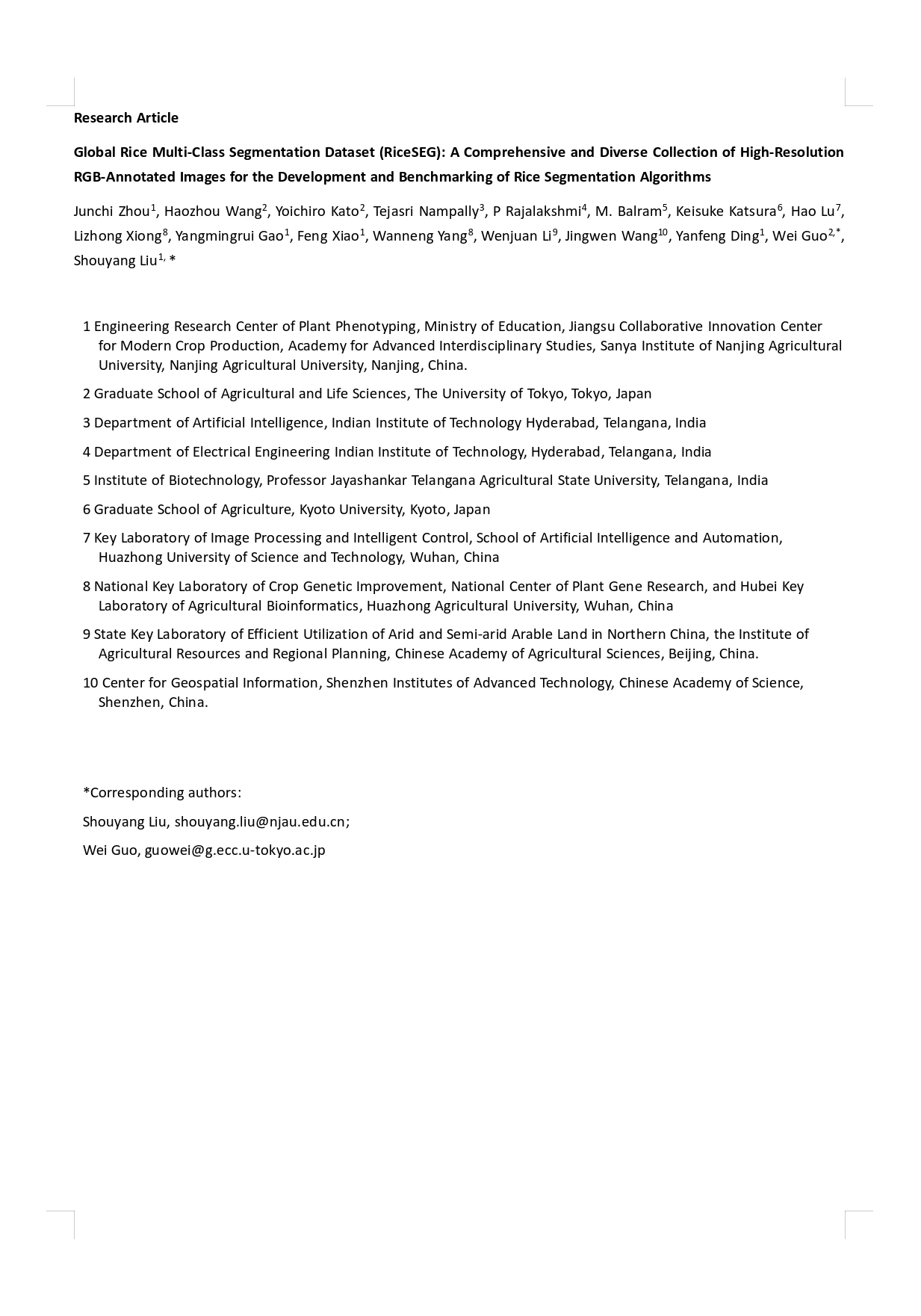